Conversational AI use cases for enterprises – IBM Blog
Today, people don’t just prefer instant communication; they expect it. Conversational artificial intelligence (AI) leads the charge in breaking down barriers between businesses and their audiences. This class of AI-based tools, including chatbots and virtual assistants, enables seamless, human-like and personalized exchanges.
Beyond the simplistic chat bubble of conversational AI lies a complex blend of technologies, with natural language processing (NLP) taking center stage. NLP translates the user’s words into machine actions, enabling machines to understand and respond to customer inquiries accurately. This sophisticated foundation propels conversational AI from a futuristic concept to a practical solution.
Several natural language subprocesses within NLP work collaboratively to create conversational AI. For example, natural language understanding (NLU) focuses on comprehension, enabling systems to grasp the context, sentiment and intent behind user messages. Enterprises can use NLU to offer personalized experiences for their users at scale and meet customer needs without human intervention.
Natural language generation (NLG) complements this by enabling AI to generate human-like responses. NLG allows conversational AI chatbots to provide relevant, engaging and natural-sounding answers. The emergence of NLG has dramatically improved the quality of automated customer service tools, making interactions more pleasant for users, and reducing reliance on human agents for routine inquiries.
Machine learning (ML) and deep learning (DL) form the foundation of conversational AI development. ML algorithms understand language in the NLU subprocesses and generate human language within the NLG subprocesses. In addition, ML techniques power tasks like speech recognition, text classification, sentiment analysis and entity recognition. These are crucial for enabling conversational AI systems to understand user queries and intents, and to generate appropriate responses.
DL, a subset of ML, excels at understanding context and generating human-like responses. DL models can improve over time through further training and exposure to more data. When a user sends a message, the system uses NLP to parse and understand the input, often by using DL models to grasp the nuances and intent.
Predictive analytics integrates with NLP, ML and DL to enhance decision-making capabilities, extract insights, and use historical data to forecast future behavior, preferences and trends. ML and DL lie at the core of predictive analytics, enabling models to learn from data, identify patterns and make predictions about future events.
These technologies enable systems to interact, learn from interactions, adapt and become more efficient. Organizations across industries increasingly benefit from sophisticated automation that better handles complex queries and predicts user needs. In conversational AI, this translates to organizations’ ability to make data-driven decisions aligning with customer expectations and the state of the market.
Conversational AI represents more than an advancement in automated messaging or voice-activated applications. It signifies a shift in human-digital interaction, offering enterprises innovative ways to engage with their audience, optimize operations, and further personalize their customer experience.
The value of conversational AI
According to Allied market research (link resides outside IBM.com), the conversational AI market is projected to reach USD 32.6 billion by 2030. This growth trend reflects mounting excitement around conversational AI technology, especially in today’s business landscape, where customer service is more critical than ever. After all, conversational AI provides an always-on portal for engagement across various domains and channels in a global 24-hour business world.
In human resources (HR), the technology efficiently handles routine inquiries and engages in conversation. In customer service, conversational AI apps can identify issues beyond their scope and redirect customers to live contact center staff in real time, allowing human agents to focus solely on more complex customer interactions. When incorporating speech recognition, sentiment analysis and dialogue management, conversational AI can respond more accurately to customer needs.
Distinguishing between chatbots, conversational AI and virtual assistants
AI chatbots and virtual assistants represent two distinct types of conversational AI. Traditional chatbots, predominantly rule-based and confined to their scripts, restrict their ability to handle tasks beyond predefined parameters. Additionally, their reliance on a chat interface and a menu-based structure hinders them from providing helpful responses to unique customer queries and requests.
There are two main types of chatbots:
- AI-powered chatbots: Use advanced technologies to efficiently address basic queries, saving time and enhancing customer service efficiency.
- Rule-based chatbots: Also known as decision-tree or script-driven bots, they follow preprogrammed protocols and generate responses based on predefined rules. Optimal for handling repetitive, straightforward queries, they are best suited for businesses with simpler customer interaction requirements.
In contrast, a virtual assistant is a sophisticated program that comprehends natural language voice commands and executes tasks for the user. Well-known examples of virtual assistants include Apple’s Siri, Amazon Alexa and Google Assistant, primarily used for personal assistance, home automation, and delivering user-specific information or services. While organizations can integrate conversational AI into numerous systems, such as customer support bots or virtual agents for companies, virtual assistants are typically used to offer tailored assistance and information to individual users.
What makes a good AI conversationalist?
Combining ML and NLP transforms conversational AI from a simple question-answering machine into a program capable of more deeply engaging humans and solving problems. Sophisticated ML algorithms drive the intelligence behind conversational AI, enabling it to learn and enhance its capabilities through experience. These algorithms analyze patterns in data, adapt to new inputs, and refine their responses over time, making interactions with users more fluid and natural.
NLP and DL are integral components of conversational AI platforms, with each playing a unique role in processing and understanding human language. NLP focuses on interpreting the intricacies of language, such as syntax and semantics, and the subtleties of human dialogue. It equips conversational AI with the capability to grasp the intent behind user inputs and detect nuances in tone, enabling contextually relevant and appropriately phrased responses.
DL enhances this process by enabling models to learn from vast amounts of data, mimicking how humans understand and generate language. This synergy between NLP and DL allows conversational AI to generate remarkably human-like conversations by accurately replicating the complexity and variability of human language.
The integration of these technologies extends beyond reactive communication. Conversational AI uses insights from past interactions to predict user needs and preferences. This predictive capability enables the system to directly respond to inquiries and proactively initiate conversations, suggest relevant information, or offer advice before the user explicitly asks. For example, a chat bubble might inquire if a user needs assistance while browsing a brand’s website frequently asked questions (FAQs) section. These proactive interactions represent a shift from merely reactive systems to intelligent assistants that anticipate and address user needs.
Popular real-world industry uses of conversational AI
There is no shortage of examples of conversational AI. Its ubiquity is a testament to its effectiveness, and the versatility of its application has forever altered how the following domains operate daily:
1. Customer service:
Conversational AI enhances customer service chatbots on the front line of customer interactions, achieving substantial cost savings and enhancing customer engagement. Businesses integrate conversational AI solutions into their contact centers and customer support portals.
Conversational AI enhances customer self-service options directly, leading to a more personalized and efficient support experience. It significantly reduces wait times typically associated with traditional call centers by providing instant responses. The technology’s ability to adapt and learn from interactions further refines customer support metrics, including response time, accuracy of information provided, customer satisfaction and problem-resolution efficiency. These AI-driven systems can manage a customer journey from routine inquiries to addressing more complex and data-sensitive tasks.
By rapidly analyzing customer queries, AI can answer questions and deliver accurate and appropriate responses, helping to ensure that customers receive relevant information and agents don’t have to spend time on routine tasks. If a query surpasses the bot’s capabilities, these AI systems can route the issue to live agents who are better equipped to handle intricate, nuanced customer interactions.
Integrating conversational AI tools into customer relationship management systems allow AI to draw from customer history and provide tailored advice and solutions unique to each customer. AI bots provide round-the-clock service, helping to ensure that customer queries receive attention at any time, regardless of high volume or peak call times; customer service does not suffer.
2. Marketing and sales:
Conversational AI has become an invaluable tool for data collection. It assists customers and gathers crucial customer data during interactions to convert potential customers into active ones. This data can be used to better understand customer preferences and tailor marketing strategies accordingly. It aids businesses in gathering and analyzing data to inform strategic decisions. Evaluating customer sentiments, identifying common user requests, and collating customer feedback provide valuable insights that support data-driven decision-making.
3. HR and internal processes:
Conversational AI applications streamline HR operations by addressing FAQs quickly, facilitating smooth and personalized employee onboarding, and enhancing employee training programs. Also, conversational AI systems can manage and categorize support tickets, prioritizing them based on urgency and relevance.
4. Retail:
Customers can manage their entire shopping experience online—from placing orders to handling shipping, changes, cancellations, returns and even accessing customer support—all without human interaction. In the back end, these platforms enhance inventory management and track stock to help retailers maintain an optimal inventory balance.
When conversational AI applications interact with customers, they also gather data that provides valuable insights about those customers. The AI can assist customers in finding and purchasing items swiftly, often with suggestions tailored to their preferences and past behavior. This improves the shopping experience and positively influences customer engagement, retention and conversion rates. In e-commerce, this capability can significantly reduce cart abandonment by helping customers make informed decisions quickly.
5. Banking and financial services:
AI-driven solutions are making banking more accessible and secure, from assisting customers with routine transactions to providing financial advice and immediate fraud detection.
6. Social media:
Conversational AI can engage users on social media in real-time through AI assistants, respond to comments, or interact in direct messages. AI platforms can analyze user data and interactions to offer tailored product recommendations, content, or responses that align with the user’s preferences and past behavior. AI tools gather data from social media campaigns, analyze their performance, and glean insights to help brands understand the effectiveness of their campaigns, audience engagement levels, and how they can improve future strategies.
7. Multipurpose:
Generative AI applications like ChatGPT and Gemini (previously Bard) showcase the versatility of conversational AI. In these systems, conversational AI trains on massive data sets known as large language models, allowing them to create content, retrieve specific information, translate languages, and offer problem-solving insights for complex issues.
Conversational AI is also making significant strides in other industries such as education, insurance and travel. In these sectors, the technology enhances user engagement, streamlines service delivery, and optimizes operational efficiency. Integrating conversational AI into the Internet of Things (IoT) also offers vast possibilities, enabling more intelligent and interactive environments through seamless communication between connected devices.
Best practices for implementing conversational AI in your business
Integrating conversational AI into your business offers a reliable approach to enhancing customer interactions and streamlining operations. The key to a successful deployment lies in strategically and thoughtfully implementing the process.
- When you implement conversational AI in your business, focusing on the use case that best aligns with your organization’s needs and effectively addresses a specific problem is crucial. Identifying the proper use case helps to ensure that your conversational AI initiative adds tangible value to your business operations or customer experience.
- Exploring different types of conversational AI applications and understanding how they can fit into your business model is vital in the early stages. This step is crucial for aligning AI capabilities with your business goals.
- Prioritizing tracking metrics accurately measures the success of your implementation. Key performance indicators such as user engagement, resolution rate, and customer satisfaction can provide insights into the effectiveness of the AI solution.
- Clean data is fundamental for training your AI. The quality of data fed into your AI system directly impacts its learning and accuracy. Helping to ensure that the data is relevant, comprehensive, and free from biases is crucial for practical AI training.
- AI training is a continuous process. Regularly updating the AI with new data and feedback helps refine its responses and improve its interaction capabilities. This ongoing training is essential for keeping the AI relevant and effective.
- Thoroughly testing the AI system before full deployment is critical. This step helps identify any issues or areas for improvement and helps to ensure that the AI functions as intended.
- Involving the organization in the implementation process, including training employees and aligning the AI initiative with business processes, helps to ensure organizational support for the AI project.
- When you choose the right platform for your conversational AI, make sure your choice is scalable, secure and compatible with existing systems. It should also provide the necessary tools and support for developing and maintaining your AI solution.
- Finally, your conversational AI’s long-term success relies on essential post-production support. This support involves regularly maintaining, updating, and troubleshooting to help ensure the AI operates effectively and evolves with your business needs.
The future of conversational AI
Based on current trends and technological advancements, we can anticipate several developments in the next five years:
- Enhanced natural language understanding: We can expect significant improvements in understanding and processing natural language, leading to more nuanced and context-aware interactions. AI will increasingly make conversations indistinguishable from those with humans.
- Personalization: Conversational AI will likely surge in personalization capabilities. By using data analytics and ML, these systems will provide highly individualized experiences, tailoring responses based on a user’s past interactions, preferences and behavior patterns.
- Increased integration and ubiquity: Conversational AI will seamlessly integrate into our daily lives more. Its presence will be widespread, making interactions with technology more natural and intuitive, from smart homes and cars to public services and healthcare.
- Advances in voice technology: Voice-based conversational AI will significantly advance. Improvements in speech recognition and generation will lead to more fluid and accurate voice interactions, expanding the use of voice assistants in diverse fields.
- Emotional intelligence: The next frontier for conversational AI involves developing emotional intelligence. AI systems will likely improve at detecting and appropriately responding to human emotions, making interactions more empathetic and engaging.
- Expansion in business applications: In the business world, Conversational AI will play a critical role in various business sectors such as customer service, sales, marketing and HR. Its ability to automate and enhance customer interactions, gather insights and support decision-making will make it an indispensable business tool.
- Ethical and privacy considerations: As conversational AI becomes more advanced and widespread, ethical and privacy concerns will become more prominent. This will likely lead to increased regulation and the development of ethical guidelines for AI development and use.
- Multilingual and cross-cultural capabilities: Conversational AI will improve its ability to interact in multiple languages and adapt to cultural contexts, making these systems more globally accessible and practical.
- Healthcare applications: In healthcare, conversational AI could play a crucial role in diagnostics, patient care, mental health and personalized medicine, offering support and information to patients and healthcare providers.
- Educational and training tools: Conversational AI will be widely used in educational learning experiences, tutoring and training. Its ability to adapt to individual learning styles and pace can revolutionize educational methodologies.
As conversational AI continues to evolve, several key trends are emerging that promise to significantly enhance how these technologies interact with users and integrate into our daily lives.
- Improved NLP: Advancements in NLP techniques like sentiment analysis and sarcasm detection allow conversational AI to better understand the intent and emotions behind user input, leading to more natural and engaging interactions.
- Cross-modal integration: Combining conversational AI with other technologies like computer vision and voice recognition will facilitate richer, more personalized interactions. Imagine a virtual assistant that can understand the objects in your room and incorporate them in its responses or adjust its tone based on your emotional state.
- In-house AI departments: As AI adoption increases, cloud pricing rises. Many organizations are bringing AI capabilities in-house to manage costs and gain flexibility, as opposed to relying solely on cloud providers to handle most of conversational AI’s computing load. Some departments may dedicate themselves to research and development, while others may focus on applying AI to specific business problems.
Evolving societal needs and expectations
The landscape of conversational AI is rapidly evolving, driven by key factors that shape its future development and adoption:
- Growing demand for AI assistants: As we increasingly rely on technology in our daily lives, the demand for intelligent assistants capable of handling various tasks and conversations will continue to grow.
- Emphasis on user experience: Developers will prioritize creating AI that not only functions well but also provides an enjoyable interaction experience. This may involve incorporating humor, empathy and creativity into AI personalities.
- Ethical considerations: As AI becomes more powerful, there will be a greater focus on developing ethical guidelines and helping to ensure the responsible use of conversational AI.
However, there are also potential challenges and limitations to consider:
- Data bias: AI models rely on data provided by humans, which can be biased in various ways. Ensuring fairness and inclusivity in conversational AI is crucial.
- Explainability and trust: Understanding how AI models arrive at their outputs will build trust and confidence in their capabilities.
- Safety and security: Robust security measures are necessary to prevent malicious actors from manipulating or compromising conversational AI systems.
As organizations navigate the complexities and opportunities presented by conversational AI, they cannot overstate the importance of choosing a robust, intelligent platform. Businesses need a sophisticated, scalable solution to enhance customer engagement and streamline operations. Discover how IBM watsonx™ Assistant can elevate your conversational AI strategy and take the first step toward revolutionizing your customer service experience.
Experience the power of watsonx Assistant
Was this article helpful?
YesNo
More from Artificial intelligence

February 22, 2024
Operationalizing responsible AI principles for defense
7 min read – Artificial intelligence (AI) is transforming society, including the very character of national security. Recognizing this, the Department of Defense (DoD) launched the Joint Artificial Intelligence Center (JAIC) in 2019, the predecessor to the Chief Digital and Artificial Intelligence Office (CDAO), to develop AI solutions that build competitive military advantage, conditions for human-centric AI adoption, and the agility of DoD operations. However, the roadblocks to scaling, adopting, and realizing the full potential of AI in the DoD are similar to those…
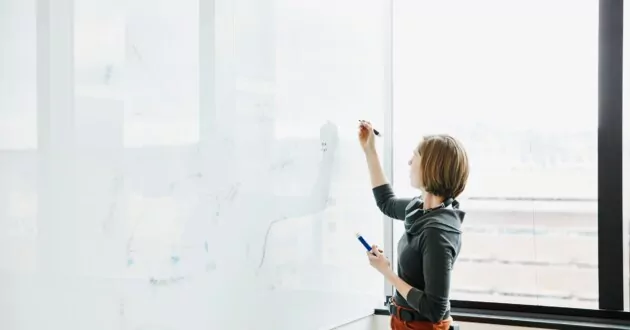
February 19, 2024
Unlocking financial benefits through data monetization
6 min read – Data monetization empowers organizations to use their data assets and artificial intelligence (AI) capabilities to create tangible economic value. This value exchange system uses data products to enhance business performance, gain a competitive advantage, and address industry challenges in response to market demand. Financial benefits include increased revenue through the creation of adjacent industry business models, accessing new markets to establish more revenue streams, and growing existing revenue. Cost optimization can be achieved through a combination of productivity enhancements, infrastructure…
February 16, 2024
Reducing defects and downtime with AI-enabled automated inspections
3 min read – A large, multinational automobile manufacturer responsible for producing millions of vehicles annually, engaged with IBM to streamline their manufacturing processes with seamless, automated inspections driven by real-time data and artificial intelligence (AI). As an automobile manufacturer, our client has an inherent duty to provide high-quality products. Ideally, they need to discover and fix any defects well before the automobile reaches the consumer. These defects are often expensive, difficult to identify and present a myriad of significant risks to customer satisfaction.…
IBM Newsletters
Get our newsletters and topic updates that deliver the latest thought leadership and insights on emerging trends.
Subscribe now
More newsletters
This post was originally published on 3rd party site mentioned in the title of this site